An AI tool for generating academic staff profiles using a pre-trained LLM – findings from a study
As part of the Role of Profiles project, the UX team learned from the work of Safa Alsalman, who investigated using AI to produce biographical content for academic staff while working for Edinburgh Innovations.
In 2023 Safa Alsalman, a student of the School of Informatics, carried out a study for Edinburgh Innovations (EI) to design and develop a user-centric tool (‘Profilify’) to automate the creation of academic profiles (or biographies) to share with EI external partners, using multiple sources. The tool was designed to be used by EI Business Development Executives (BDEs) who typically carry out this process without AI intervention. Safa’s study involved user-centred design of the tool, informed by consultations with different BDEs, followed by evaluation of the biographies generated by the tool (evaluations were done by the BDEs and by EI external partners).
Safa’s written account of the study has been very helpful for the team to consider in light of our project goal of shaping recommendations to improve the provision for staff profiles at the University. Some of the things we learned from reading her work, together with potential implications for our project are detailed below.
The study provided insight into how Edinburgh Innovations use profile content
In the pre-design phase of her study, Safa interviewed Business Development Executives from across the University to understand the process they carried out to compile academic profiles and biographies from the sources available, for use by external partners of Edinburgh Innovations. In these interviews several themes emerged, giving insight into what makes a compelling academic profile and the difficulties associated with compiling them.
Building compelling academic biographies depended on content from multiple sources
When they were tasked with pulling together a profile for a named academic or to seek an appropriate academic for a specialist area, BDEs typically begun by gathering existing sources of profile information. The University’s Research Explorer was their primary source, however, Google Scholar was another important go-to for information about academics’ work. Other sources included word-of-mouth from colleagues, School websites, LinkedIn and Opportunity Match (an AI-driven search engine developed by the University to enable people at the University to find academic expertise and make connections).
Read more about Opportunity Match:
Opportunity Match page on the Bayes Centre website
Inconsistently structured profile content made it difficult to compile good academic biographies
Commenting on their process to create academic biographies, the BDEs noted several difficulties using source information from Research Explorer including:
- Insufficient or inadequate information about the academic in question (for example, empty overview sections)
- Outdated information (for example, information about staff members who had left the University)
- Non-uniform structure or quality of information (for example, some overviews contained a short sentence whereas others contained structured paragraphs, and some were written in the first person, with others written in the third person)
- Content not relevant for biographies for external partners (for example, advertising for PhD candidates).
Projects and recent work were particularly important to include in academic biographies
The BDEs were asked to consider the different pieces of information that could be included in an academic biography and asked to rank these in order of importance (bearing in mind the interests of external partners who would access the biographies). The pieces of information included:
- Name
- Image
- Job title
- Contact information
- Social media accounts
- Affiliation
- Position
- Education
- Past career
- Research information
- Projects
- h-index (a measure of how impactful a piece of academic work is)
- Citations
- Most cited work
- Most recent work
Name was unsurprisingly ranked of highest importance. Some BDEs felt job titles were important, whereas others deemed them less so. Images were also ranked as important by most BDEs who felt they were useful to give a visual sense of the person external partners may be working with. The majority of BDEs felt that projects and most recent work were of high importance, considering external partners to be interested in present-day activities and the potential application of relevant research activities to commercial realms. Opinions on the importance of social media accounts were mixed, with some BDEs considering social media (particularly LinkedIn) to be a good way to promote academics’ work.
There was a need for better tools to manage and compile profile data
When interviewed, the BDEs said they would have appreciated better tools to help them decide which information to include in academic biographies, manage academic information and maintain and disseminate information about academics. These insights helped inform the design and creation of prototypes for the automated tool for BDEs to use.
Six requirements formed a blueprint for a tool to build biographies
Thematic analysis of the data from the BDE interviews helped define specific requirements for the profile-building tool. These were as follows:
- Functionality to generate customisable profiles, tailored for client preferences
- Ability to produce profiles resembling a CV for a professional academic representation
- Incorporation of tag lists to highlight research themes within the profiles
- Use of tabbed menus for projects and publications
- Implementation of a mechanism for verifying profiles
- Inclusion of a concise summary of the academic’s background
Designing the automated tool highlighted two ways to build academic biographies
In the design and evaluation phase of the study, BDEs were engaged to provide formative evaluative information to guide tool design.
The tool could build biographies following an additive or a subtractive process
Mass-market website building software informed the tool design. This software typically offers two approaches to constructing websites:
- Starting with a blank canvas and building up from scratch (additive)
- Using a pre-defined template and removing unnecessary elements (subtractive)
To investigate which approach was preferable from the BDE user perspective, one prototype to represent each was built to be evaluated.
Each prototype started by guiding the BDE user to find sources of information about their academic of interest by typing the name of the academic into a search bar. Subsequently, they were directed through a series of steps to build up the profile from the information gathered. In the additive prototype, the user was directed to different pieces of information to decide whether to include it or exclude it, whereas in the subtractive version, they were presented with a fully populated profile to decide which pieces of information should be removed.
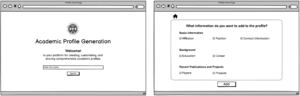
Screenshot of prototype of automated tool to build profile content in an additive way
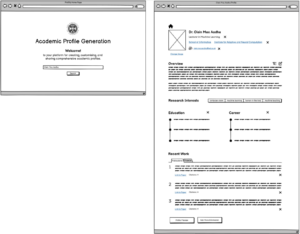
Screenshot of prototype of automated tool to build profile content in a subtractive way
In later stages of the study, the BDE users had the option to preview the profile, finetune it and to verify it with the academic in question before sharing with external partners. When they tried out both versions of the tool, they were able to use both types to successfully generate profiles and there was no clear preference for additive over subtractive.
Building the automated tool required multiple design decisions
The tool needed to satisfy the needs of two sets of users, firstly the BDEs (who would use it to assist them in building profiles) and secondly the external partners (who would consume the output – the biography content generated by the tool). Meeting the needs of both audiences drove design decisions.
One such decision was to develop AI Agents to draw in data from sources in addition to those from the University (including Google Scholar, Google Search, Google Images and YouTube) – to ensure sufficiency of profile data.
Another decision was to select the LLM gpt-3.5-turbo for the tool – based on its proficiency for high accuracy in summarisation (established according to the Holistic Evaluation of Language Models (HELM) benchmark) – to ensure high-quality outputs from the tool.
Read more about the HELM benchmark for LLMs on the Stanford University website
Content in the profiles generated by the AI tool was 78% accurate
The tool was used to create profiles for 15 academic staff, and BDEs and external partners assessed the accuracy of the content in the resultant profile. They found that, across the 15 profiles there was a 78% accuracy rate.
Some pieces of automated biography content were more accurate than others
On the whole, the AI tool had performed well in including the correct images, homepages, research themes and publications. It had not performed as well in providing up-to-date links to relevant social media accounts and in some cases it had failed to distinguish between multiple academics with the same name.
Academic number | Academic initials | Image | Homepage | Overview | Research Themes | Publications | ||
1 | M.B. | accurate | accurate | accurate | accurate | accurate | accurate | accurate |
2 | M.R | correct | accurate | accurate | accurate | accurate | accurate | accurate |
3 | S.S. | correct | accurate | accurate | X | accurate | X | accurate |
4 | I.M. | X | accurate | X | accurate | Unverifiable information | accurate | accurate |
5 | O.M. | accurate | accurate | accurate | accurate | accurate | accurate | accurate |
6 | N.G. | accurate | accurate | accurate | accurate | Wrong name | accurate | accurate |
7 | D.A. | accurate | accurate | X | Wrong name | accurate | accurate | accurate |
8 | L.B. | accurate | accurate | accurate | accurate | Unverifiable information | accurate | accurate |
9 | J.C. | accurate | X | accurate | accurate | accurate | X | X |
10 | E.I. | accurate | accurate | accurate | accurate | accurate | accurate | accurate |
11 | G.I. | accurate | X | X | X | X | accurate | accurate |
12 | K.E. | accurate | accurate | accurate | X | accurate | accurate | accurate |
13 | M.I. | accurate | accurate | accurate | X | accurate | accurate | accurate |
14 | B.R. | accurate | accurate | accurate | accurate | accurate | accurate | accurate |
15 | P.B. | accurate | Broken | accurate | X | accurate | X | X |
Total | 14 | 12 | 12 | 9 | 11 | 12 | 12 |
Table to show which information the BDEs deemed to be correct in academic biographies generated by the tool
The tool was deemed usable by staff testing it
Reviewing the usability of the tool with the BDEs, it was well-received and was rated ‘excellent’ on the System Usability Score index, indicating that they deemed the tool both usable and useful to help them produce biographies of academic staff.
Implications for The Role of Profiles project
Details from Safa’s study have helped us take a fresh perspective on what is important for a future provision for University staff profiles, and to consider the potential to apply AI to profile content management.
University staff profiles are only one source of data about academic staff
As Safa’s study found, academic staff working at the University may have information about themselves published in multiple locations, therefore any improved provision for profiles in EdWeb2 will sit alongside other sources, and depending on the needs of audiences for this content, certain sources may be more preferable than others (for example, profile content in Google Scholar may be more familiar to external partners or more likely to be returned in an internet search than content in University systems such as Research Explorer).
Reiterated importance of well-structured, up-to-date profile content
The difficulties the BDEs shared relating to making sense of unstructured content when compiling academic biographies suggested a more templated approach to profiles (including tagging features for cross-referencing areas like research themes) would help end-users make sense of profile data. Furthermore, structured profile content would also support better indexing of content, to facilitate more flexible search approaches including the increasing use of AI-powered search approaches to find information. The importance the BDEs placed on content about recent activities of academics (for use by external partners) strengthened the case for finding ways to make it easier for academics to keep their profiles up-to-date.
Profiles can be built in additive or subtractive ways
Comparing staff evaluations of the additive and subtractive prototypes of the profile-building tool showed that both versions were capable of successfully producing profiles, and that some BDEs preferred additive, some subtractive. It is likely that a similar split in preference may be found with University staff when it comes to building their profiles – where some prefer the ‘blank canvas’ approach, others are likely to want a ‘templated’ approach. Any future profiles provision may need to cater for both sorts of preference, however, should include the ability to structure content to enable findability benefits.
LLMs can help generate profile content, but human verification is needed
Evaluation of the ‘Profilify’ tool by the BDEs and its outputs by BDEs and external partners showed it was useful and usable, and was capable of producing reasonably accurate biographies. Taken together, the results of Safa’s study indicated an opportunity to incorporate use of an LLM into workflows to generate n of academic biographies, therefore potentially saving manual work for staff. As with any LLM intervention, however, it is important to consider that outputs are unlikely to be 100% accurate and/or suitable for context, suggesting that while LLMs could be part of the solution for an improved profile provision, they are unlikely to be the entire solution.
Potential for AI agents as a tool to automate tasks and tailor profile content
Drawing on this study as well as our own team’s previous experimentation with Drupal AI Assistants in relation to search and content preparation it was possible to recognise several possible ways LLM-powered AI Agents could be applied to staff profile generation, for example:
- AI Agents could be deployed to carry out specific stages of the process to compile a profile, for example: to structure content into defined sections, to check content matched the rules of the University’s Editorial Style Guide
- Through tailored prompt augmentation, AI Agents could be developed to write profile content for specific audiences, for example external partners with particular interests
As with the Profilify tool, decisions about the individual component parts of an AI mechanism, including the input data sources and the specific LLM would require careful consideration based on the needs of profile builders and end-users of profile content.
Read more about the AI experimentation from the UX team:
Testing ELM’s ability to return useful results with prompts about the Editorial Style Guide
Can AI help or hinder search? Trials with Drupal AI-boosted search and AI Assistants
An automated Editorial Style Guide? Experimenting with Drupal AI Automators