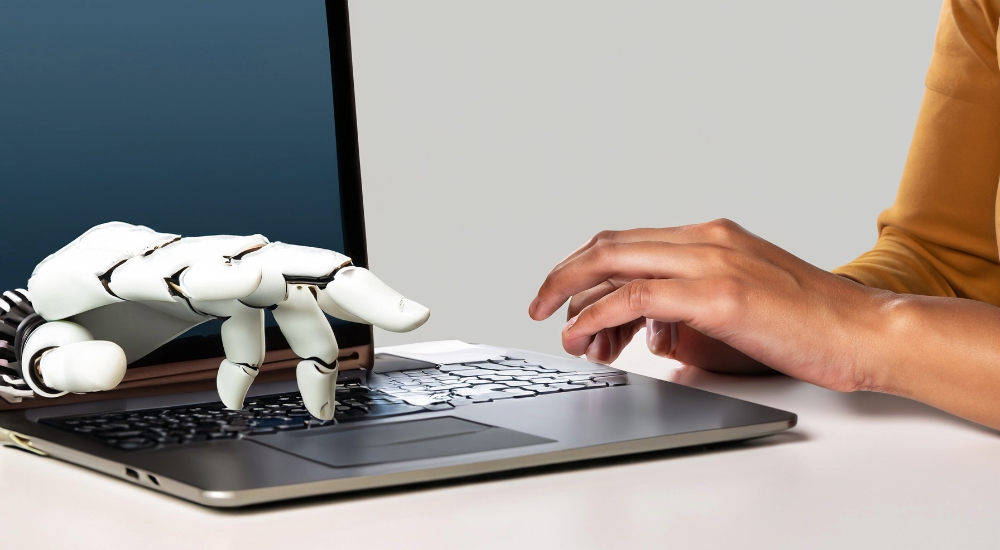
Where were we?
Yes, Gen-AI is a storm that impacts higher education!
My personal interest started in late November by playing around with a couple of questions and related sources. Primarily, I have been aware of the related APIs for different tasks since those days, but I was not aware of the big change. After spending some time with silly prompts, I started to think about my courses and related teaching materials. It feels like, what about asking a specific question from my course assessment or a potential improvement of course delivery? This pushed me to do more reasonable experiments relying on certain tasks, questions, and structured prompts. In the meantime, it was important to dive into the heart of such models to see what was really happening.
‘’There is an opportunity to refocus how we assess learning away from the ability to produce well-written essays towards ‘more sophisticated things like comparison, critique, adaptation, refinement’ ‘’ (Victor Lee, Stanford University) [1]↗️
Going back to my experiments, it was very useful in carefully used cases, including my efforts to write good prompts and tune the generated output by giving more specific inputs. Not surprisingly, such tools are not calculators, just language models, so we need further fact-checking, especially if the correctness of the answer is the most important aspect. On the other hand, when the need for correctness is reduced, it can be very beneficial to create generic texts to inspire from and adjust based on our needs. As one of my use cases, I could write my feedback on presentations very quickly while different teaching duties were happening. In another use case, by considering my questions and solutions, it was easy to create structured and grammatically concise rubric draft. Finally, creating our own knowledge base systems based on our own text data seems very promising in the longer term. Even the small experiments that I conduct indicate the certain potential of the potential advent tutoring bots.
“Our own hope is that, through AI, we can eventually approximate a 1:1 teacher [to] student ratio for every student in CS50, as by providing them with software-based tools that, 24/7, can support their learning at a pace and in a style that works best for them individually” (David J. Malan, Harvard University) [2]↗️
Another parenthesis should be opened for the data science pipeline, as many practitioners have mentioned recently. Regarding the released tools like Chat-GPT Code Interpreter, the already existing CoPilot, and the one Stack Exchange announced as OverflowAI, the way we code or learn coding is under the impact of all such tools [3]↗️. In that respect, we cannot avoid the use of such tools in the long term, whereas the industry has already started to look for such skills in specific jobs. However, as another big challenge, the assessment approaches or question design that we followed so far should be revisited by knowing the capabilities and limitations of Gen-AI-enabled tools and their potential future. One of the potentials of open-source models is that they may allow us to use such tools in a more personalized way based on our needs and our own data soon.
‘’It sounds ridiculously science fiction, but there is a world in which everyone has their own ongoing AI assistant’’ (Simon Willison, Independent Researcher) [1]↗️
When we change our camera lens from higher education to society, it is crucial to highlight that the potential risks of such Gen-AI-based tools must be a global priority, and it certainly requires a worldwide discussion tied to certain collaborative efforts. Remembering the fact that we did not use this chance during the pandemic period not a long time ago, personally, I am suspicious about finding a fruitful environment to prioritize all human beings rather than falling into a nationwide competition. Certainly, there are real potential risks that can affect overall society beyond the impacts on higher education, such as environmental issues, threats to cybersecurity, the spread of misinformation and disinformation, and pumping out more material riddled with bias and fabrications. Let us say, revolutionizing the future of businesses should not be the only topic on our worldwide agenda.
‘’The worry about polluting the information ecosystem is real. The one thing that we should have learned from Trumpism, and the pandemic is that our civilizations are more vulnerable than we think’’ (Stephen cave, University of Cambridge) [1]↗️
There are various related questions that we can ask and wait to find a good answer to. Besides, Gen-AI is still in its early stages and has plenty of space to investigate, but it can still be handy whenever it is used wisely. Specifically, going back to the higher education ecosystem, as a young educator, I feel that we need to collaborate with students at a certain level so that we can both learn from each other and guide them efficiently. Rather than leaving them in a huge playground without any map, the rise of Gen-AI and its potential impacts call us to play together. If I try to use one of the interesting, well-known phrases available for young adults and their parents in Turkey in a different way;
Don’t smoke secretly from me. I’d rather you smoke where I can see it.
REFERENCES:
[1] Living with AI↗️, AI Special Report, New Scientist, 29 July 2023. [2] Harvard’s New Computer Science Teacher Is a Chatbot, available in https://uk.pcmag.com/ai/147451/harvards-new-computer-science-teacher-is-a-chatbot↗️[3] Xinming Tu, James Zou, Weijie J. Su, Linjun Zhang, 2023. What Should Data Science Education Do with Large Language Models? Available in https://arxiv.org/abs/2307.02792↗️
Ozan Evkaya
Ozan Evkaya is one of the University teachers in Statistics at the School of Mathematics↗️ at The University of Edinburgh. Previously, he has held postdoc positions at Padova University (2021) and KU Leuven (2020), after completing his PhD at Middle East Technical University in 2018. His academic curiosity lies in the fields of copulas, insurance, and environmental statistics. He is ambitious about improving my computational skills by leading, organising, or being a part of training workshops and events.
https://www.linkedin.com/in/ozanevkaya/↗️
https://twitter.com/ozanevkaya↗️
https://www.researchgate.net/profile/Ozan-Evkaya↗️