In recent years, attention has turned to the environmental, social and economic costs of designing, developing, and using AI across various industries.
Equality and inclusion has often been an afterthought in AI design and development, leading to new and exacerbated inequalities and harms in both digital and offline realms. The trend is for AI models to become larger and more expensive, which has implications for how inclusive they are for PSM of varying sizes and with differing budgets, and for their carbon footprint. PSM need to be asking questions about how they can use AI for sustainability and about the sustainability of AI.
We focused on scoping out what kinds of questions PSM need to be asking about their use of AI and data-intensive systems. These included:
- Does PSM need to re-imagine ways of working (e.g. mechanisms of governance) to create fairer and more sustainable futures for people and the planet?
- How might PSM ensure oversight of their AI-related supply chains with regard to environmental sustainability and fair working practices?
- What are best practice examples from which PSM can learn – and what initiatives currently exist within PSM?
- Which AI applications or approaches may be most appropriate/beneficial and which may exacerbate inequalities and harms?
- Is there a role for PSM to use AI to better editorially cover these issues? Or might this create contradictions with stated sustainability and equality goals?
- What research is needed to advance credible AI sustainability and equality strategies?
We identified 4 themes:
-
Data and digital poverty & structural inequalities
-
New technology is at times considered/construed as inherently good, and inequality as a side issue but equality must be put at the centre for PSM
-
There is often a focus on universality in terms of content but not in terms of infrastructure and access, and lack of recognition of digital inclusion and literacy belonging to a small, ‘digital elite’ class
-
Data and digital poverty are core concerns here
-
Often not recognised that 11m people in Britain are functionally disconnected, and another 20-30% of population are limited users (e.g. elderly, lower working class), with another group of young people who are only consuming a very small amount of targeted media
-
But you don’t have to be online for algorithms to discriminate against you
-
-
PSM should be proactively researching how new data-driven services might compound existing inequalities, e.g. exacerbating digital/data literacy issues, excluding communities, under/mis-representing people/groups
-
Digital inequality is driven by the same variables as other inequalities, including health, education, socio-economic variables – is there a risk some of these fundamental questions are eclipsed as they’re seen as ‘boring’ compared to hot topics in society such as bias in police systems/grants & loan allowances etc?
-
-
What more can be done in the regulation and policy space to influence this agenda?
-
E.g. PSM have argued for more transparency in data sets (e.g. for training ML) and for data/dataset access for public bodies
-
-
Big question for PSM here is where they derive their conceptual models of ‘good’ AI/data-driven systems and services
-
Adopting from/aping commercial industry won’t work
-
It is expensive and resource-intensive to make these systems work for everyone (principles of universality, inclusion)
-
-
Public discourse & digital literacies
-
Most commercial data-driven systems cater to a targeted market and can ignore groups (e.g. disconnected, rural, elderly) where the systems are not financially viable – PSM cannot
-
So there is a question as to whether PSM should do more to tackle the issues of digital poverty and do more in the area of digital education?
-
Particular case might be the framing of AI in the public discourse – and the role tech companies play in narratives around AI & algorithms
-
Examples exist, such as VRT in Belgium going into schools to teach children how AI actually works
-
-
Should PSM move towards a more participatory design approach where users are embedded in the research and design in order to increase digital inclusion & literacy?
-
Beyond focusing directly on users, and more specifically often early adopters, what can PSM do to improve its innovation models and methods in this regard?
-
What role can PSM play in empowering users in relation to their data to counter their feelings of (and the reality of) disempowerment, discomfort and impotence in relation to prevailing extractive data practices?
-
A good example of how to bring “expert” lay people into a process might be contemporary medical research – paid and longitudinally engaged, and incentivised through research culture (e.g. requiring those affected be part of process)
-
-
PSM being denied access to data by platform intermediaries hampers efforts to understand their audiences access, preferences etc.
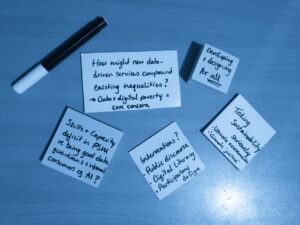
-
Taking sustainability seriously & considering climate justice
-
PSM have responsibility around environmental issues, not only of their own use of technologies (including AI) but also how they disseminate information around this and how they are complicit with creating constant demand for new materials (devices and content)
-
Carbon footprint reduction is only one measure – materials, minerals, manufacturing etc. for technologies often overlooked. Data-driven strategies are energy and resource intensive, and these resources are often being mined unethically from the global south
-
PSM must think about their role in driving desire/need for new devices and ensure compatibility for older systems, consider repair and recycling opportunities
-
-
One justification for an increasingly data-driven world is ‘efficiency’, and savings (energy, time, effort etc) and this is a key motivation for the use of AI in PSM. However, the Jevons paradox states that using a resource more efficiently leads to economic growth, which ultimately means more of that resource is used overall
-
In BBC lifecycle assessments of media consumption, the end-user has the largest proportion of energy use, however, users have far less agency in reducing their climate footprint. Should the obligation rest with corporations/governments instead?
-
There is a growing volume of public opinion on the area of climate justice and this needs to be brought into the conversation, PSM is one important way to do this
-
When talking about sustainability it is often focused on how to continue current ways of practice but in a less impactful/harmful way – but a climate justice approach posits that current practices are inherently unjust and we need to move the conversation onto creating a more just future
-
The concept of slow technology is worth investigating – asking: do we need to do it, what’s the impact of doing it, is it worth doing?
-
-
Working to make PSM good data guardians & informed consumers of AI
-
There is still a lack of understanding around AI and most public sector bodies are in position where they don’t know enough to be good guardians or informed consumers of AI technologies
-
There is still work to do on defining AI, the possibilities of AI in PSM, ethics of engaging with AI and how it can be used as a tool for inclusion and diversity.
-
The starting point for many users and other PSM organisations is much lower than for example, the BBC with its well-resourced R&D team
-
-
There is a push for public sector bodies to use their data, ‘do data science’, analytics etc. but without the same insistence and education regarding the ethics and social ramifications – or a real questioning of why it is being processed at all
-
‘Consultancy culture’ often pushes these technologies on public services when they are not informed/ready and have not identified their own motivations, goals and value frameworks for adoption
-
-
It is unhelpful when a fear of falling behind or inferiority is the driving force behind adopting technologies
-
Progress then becomes defined in narrow terms of e.g. adopting AI, VR, AR etc
-
If the question is turned around to first ask how technology can help reduce inequalities, it changes the perspective to more of a public interest focus
-
With thanks to our participants
Dr Rashné Limki
Lecturer in Work and Organisation Studies, University of Edinburgh
Dr Gemma Cassels
DDI Public Sector Lead, University of Edinburgh
Prof Simeon Yates
Associate Pro-Vice-Chancellor Research Environment and Postgraduate Research, University of Liverpool
Dr Oliver Bates
Research Fellow, Lancaster University
Prof David Kirk
Professor of Human-Computer Interaction and Director of Open Lab, Newcastle University
Lalya Gaye
AI & Data Initiative Coordinator at European Broadcasting Union
Francesca Scott
Diversity, Equity and Inclusion Officer, European Broadcasting Union
Dr Rhia Jones
Research Lead, BBC
Philip Robinson
Lead Architect, BBC