Media companies gather and produce large amounts of video, audio and textual material, including news in different languages as well as scripts and subtitles. Natural Language Processing (NLP) techniques are being used to help them deal with this abundance of data, for example to extract semantic data, classify content, and analyse sentiment. Machine translation and text-to-speech/speech-to-text systems underpin production tools and are driving new forms of delivery such as voice with the aim of personalising content and attracting new generations of audience.
In this workshop, we asked:
- How is/might NLP be used in PSM?
- What areas are ripe for exploration and development to deliver new forms of public value?
- What combination of disciplines and skillsets are needed for responsible research and innovation in this field?
We identified one overarching theme and four sub-themes:
The fundamental importance of differentiating PSM needs from the priorities of other (e.g. commercial) media and ensuring NLP research and innovation efforts clearly reflect this was our overarching theme. Four key thematic areas were identified that could help guide such a public interest-aligned research agenda:
-
Inclusivity, representation and equality
-
Inclusive NLP systems for PSM are needed to promote trust and accurately represent views/news from all communities, especially those that are currently under-represented. The same is true for interactive, e.g. voice systems.
-
There are many aspects to inclusivity, e.g. multilingualism, age, gender, disability, and focus to date has often been on language, but there is a need to broaden this out.
-
In terms of language inclusivity, there are issues still to tackle around English accent translation (regional and non-native accents), developing minority language systems (which have less training data available) and offering multilingual stories/services.
-
To train NLP models, a huge amount of data is required. There is a current focus on making models work with existing data to help reduce user data issues
-
PSM have a duty to be representative of the whole of the UK and ensure due impartiality – might NLP help identify and analyse bias in output (e.g. representation of specific societal/geographic groups, gender of voices, certain groups of thoughts)
-
Trustworthiness and the role of explanation
-
With AI/ML, often journalists will be required to use ‘black box’ models of which they have no understanding of the inner workings. They rely on the sources/systems they are using but creating explainable NLP/large language models is very difficult. This lack of explainability reduces transparency of the models and reduces trustworthiness of systems for PSM.
-
This leads to a problem of who controls what and who does the responsibility/accountability of outputs lie with – is the onus on journalists or the machines/systems they are using to be trustworthy and transparent?
-
There is a deeper issue here of definitions of the words themselves – what does transparent, explainable, trustworthy mean? In addition to being difficult to define, these concepts then have to be translated into computational form which adds another layer of difficulty. How do we build common vocabularies and bridges between disciplines?
-
Big fundamental questions remain in other key application areas like fact-checking and content verification, such as what is a ‘checkable’ fact and whether NLP can identify this in the first place or generate a ‘ground truth’ when context matters. How can we ensure NLP techniques are not misapplied?
-
This relates to questions of how models can be explained. On top of technical issues of explanation, there are social issues – to whom should the models be explained (journalists, end users), how and why?
-
To tackle issues of explainability and transparency, there is a need to co-design systems with journalists and technologists. Currently, the user is often the ‘object’ of a system, rather than the ‘subject’.
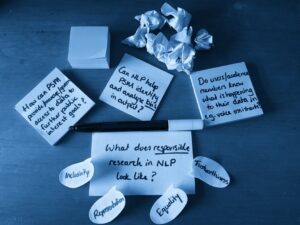
-
Personalisation in the public interest
-
A big aim of PSM is personalisation, for example through recommendation systems geared towards user preferences, some of which use NLP. Often, the success of these is measured on metrics such as click-through rate. How can we optimise systems on values beyond this which have deeper societal meaning?
-
Recommender systems often produce ‘average’ content that creates a distribution platform for fake news where the most accessed content can dominate. How can we efficiently create local content that promotes PSM ideals and is impartial and trustworthy, whilst still relevant to a particular community?
-
Can NLP help identify a person’s full range of interests from their digital interests, and should this even be attempted?
-
More research is needed into whether users know where and what is happening to their personal data, e.g. voice, speech
-
Better managing data sharing for research and public interest-focused outputs
-
How can PSM provide/manage/govern access to data to further public interest innovation goals? Big AI companies are releasing data sets and tools as a way to get researchers to focus on the problems they care most about. Could more be done in this regard in public service media around language and speech?
-
Research is needed to explore what the barriers are to data sharing with academia and other industry partners/networks (e.g. contractual/risk/reputation/cost-resource) and address them.
-
Multidisciplinary teams will be central to this effort, e.g. NLP experts collaborating with ethicists/communications/law and policy scholars.
Next steps:
Building a network
We will continue to bring researchers into conversation with industry to build a network of experts interested in ensuring AI works in the public interest in media and journalism. If you want to be kept in the loop, please contact: Bronwyn.jones@ed.ac.uk
Constructing a research agenda
We will take the insights from this workshop and build a mission-driven research agenda – identifying funding opportunities and scoping out work packages that address these pressing issues of societal significance.
With thanks to our participants:
With thanks to our participants
Dr Peter Bell
Reader in Speech Technology, University of Edinburgh
Dr Lexi Birch
Innovation Fellow at ILCC, School of Informatics, University of Edinburgh
Dr Catherine Lai
Lecturer based in the Centre for Speech Technology Research, University of Edinburgh
Dr Björn Ross
Lecturer in Computational Social Sciences, University of Edinburgh
Dr Barry Haddow
Research Fellow in the ILCC, University of Edinburgh
Dr Sondess Missaoui
Research Associate in AI, University of York
Sanne Vrijenhoek
Researcher in Faculty of Law, UV Amsterdam
Dr Andy McFarlane
Reader in Information Retrieval, City University of London
Alexandre Rouxel
Data Scientist at European Broadcasting Union
Sevi Sariisik
BBC News Labs
Hazel Morton
Voice and AI, BBC
Dr Rhia Jones
Research Lead, BBC