Past Research Projects
Using geospatial data and techniques in response to COVID-19 social distancing requirements in Bulawayo, Zimbabwe
Background
The World Health Organisation has called for social distancing as one of the methods to reduce the spread of Covid-19. Like many places, Zimbabwe has used lockdowns to curb the spread of the virus with the enforcement of social distancing and encouraging personal hygiene through regular hand washing. In low-income countries full lockdowns can often be difficult due to the close proximity of homes, little suitable outdoor space for exercise, houses that lack reliable electricity and water supplies and a reliance on informal employment. All of these mean that people have to leave their homes on a daily basis for basic supplies. SARS-CoV-2 is known to be transmitted through social contact which, in a lockdown situation, will primarily be at vending outlets which are likely to serve large numbers of people. In this project we used local government data on land cover across the city along with building footprint data from open-street map (OSM) and very high-resolution satellite imagery to map and identify potential problematic areas in the City of Bulawayo, Zimbabwe.
Method
The COVID-19 pandemic is in the process of unfolding and as such complete data relating population density to disease outbreak, transmission and morbidity are incomplete. The basic reproductive number (R0) is associated with population density regardless of transportation accessibility and median income implying the opportunity for effective contacts are mostly driven by crowding in denser areas. We calculated a density of residential buildings per hectare for each building. Using this method rather than a predefined unit of analysis allows for high density clusters of dwellings to be identified at city level and within the high-density clusters, particular high-risk buildings can also be identified. Overlaying the suburbs data gives an indication of the suburbs with high density clusters. Taking averages across suburbs and tabulating statistics on average, maximum and standard deviation at suburb level, allows for the identification of particular suburbs displaying these potentially high-risk characteristics. Feeding these density maps back to the city government we find that the high density areas are often those with poorer households that are likely to struggle to maintain social distancing easily.
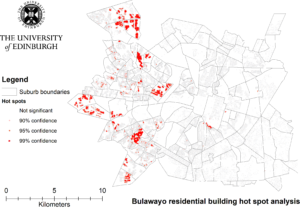
This map shows areas within suburbs that have a higher than average density of residential buildings and therefore maybe locations that are at higher risk of COVID-19 transmission.
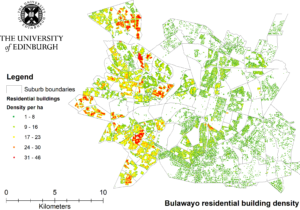
This map shows the number of residential buildings per hectare within suburbs across Bulawayo (click to enlarge image).
We further analysed the data to identify hotspots and provide confidence measures for these hotspots. Hotspots here are areas within suburbs that have a higher than average density of residential buildings in the local area and thus indicating regions or blocks of houses that are at higher risk of transmission. Overlaying this with a land cover map the government can then also identify potential areas within the city that could exacerbate the transmission such as schools, shopping areas, green spaces.
Comments are closed
Comments to this thread have been closed by the post author or by an administrator.