Artificial Intelligence: The future of rare disease research?
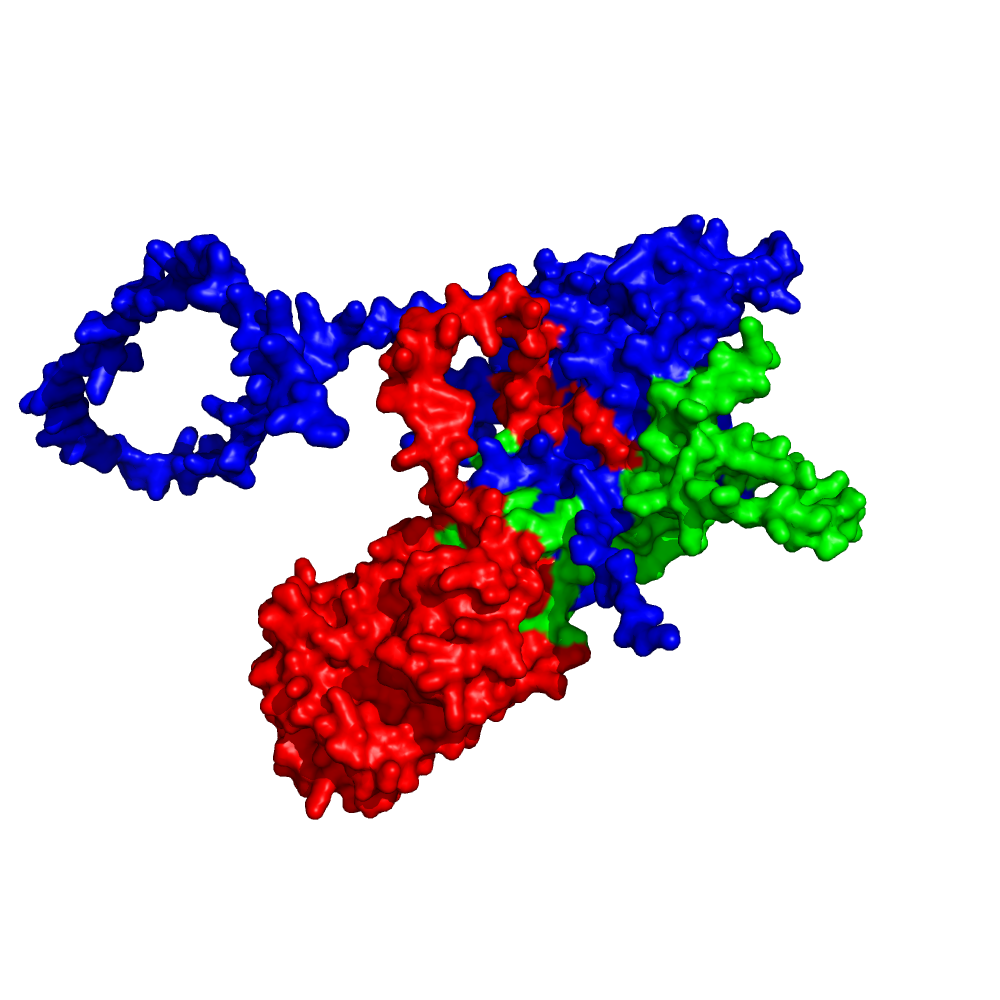
Above: RNAse H2 structure, predicted with AlphaFold-Multimer. The visualization of interfaces raises questions on mechanisms of action, which can then be experimentally tested in the wet lab.
Artificial Intelligence, or AI, is slowly starting to make its way into every aspect of life. In the increasingly data-driven field of Biology, tools like AlphaFold are revolutionizing what individual biologists can study and achieve. In rare disease, AI is replacing experimental techniques with much faster and cheaper computational predictors, and even unlocking new ways to study rare disease entirely. My name is Maarten van den Ancker, and during my PhD I would like to use AI to study rare disease in new ways.
Artificial Intelligence is, simply put, programs designed to do a task that normally requires human intelligence, like problem-solving, learning, and decision-making. AI generally accomplishes this by learning, adapting its execution and improving the overall performance from data. What this means is that the output of an AI is just a reflection of the data you put into it, so selecting high quality inputs makes it more likely to get high quality outputs.
One example where researchers at the IGC have benefited from AI to accelerate mechanistic discovery in the study of rare diseases is in the Jackson Lab. Here they worked with the Walter lab at Harvard who used AlphaFold-Multimer to screen interactions between DONSON, which is mutated in microcephalic dwarfism, and replisome proteins [1, 2]. Through this in silico screen, these researchers were able to identify a novel role for DONSON in vertebrate replisome assembly, and implicate dysfunctional replisome assembly in the pathophysiology of microcephalic dwarfism. The novel role of DONSON was identified through an AlphaFold-Multimer screen as promoting CMG assembly by helping correctly position TOPBP1 and GINS [1]. They then confirmed these interactions biochemically, and made a mouse model with a DONSON mutation found in a patient with microcephalic dwarfism, which recapitulated the microcephalic dwarfism phenotype and had defective CMG assembly, showing how AI-based predictions can accelerate discovery of disease mechanisms.
AI is only going to become more and more important in rare disease research, as our capabilities increase with every improvement of an algorithm. AI is going to be particularly helpful in rare disease research as well, since generating cell lines for hundreds of thousands of unique mutations is not feasible, but AI can screen through that many mutations in a relatively short amount of time. Luckily, there are powerful accessible platforms now available for structural prediction, for example the ColabFold-Batch notebook (https://colab.research.google.com/github/sokrypton/ColabFold/blob/main/batch/AlphaFold2_batch.ipynb) allows researchers to batch predict protein complexes using an interactive web interface [3]. I believe the work we are doing at the IGC will enable wet-lab researchers to access next generation mechanistic discovery techniques that AI enables more easily.
References:
[1] Lim Y, Tamayo-Orrego L, Schmid E, Tarnauskaite Z, Kochenova OV, Gruar R, et al. In silico protein interaction screening uncovers DONSON’s role in replication initiation. Science 2023;381. https://doi.org/10.1126/science.adi3448.
[2] preprint: Richard Evans, Michael O’Neill, Alexander Pritzel, Natasha Antropova, Andrew Senior, et al. Protein complex prediction with AlphaFold-Multimer. bioRxiv 2021.10.04.463034; doi: https://doi.org/10.1101/2021.10.04.463034
[3] Mirdita M, Schütze K, Moriwaki Y, Heo L, Ovchinnikov S, Steinegger M. ColabFold: making protein folding accessible to all. Nat Methods 2022;19:679–82. https://doi.org/10.1038/s41592-022-01488-1.