Given its potential, why would anyone argue against personalised learning?
(Personalization vs Personalized learning) has shifted the center of attention from the impact of quality teaching, to the power of a non-human classroom.
– Arantes (2023)
Personalised Learning vs. Personalisation
The debate around personalised learning often stems from the conflation of personalised learning—mainly a human-led process—and personalisation, which is AI-led and data-driven. It is essential to differentiate the two before examining why personalised learning, despite its benefits, might face resistance (Arantes 2024).
PERSONALISED LEARNING | PERSONALISATION |
---|---|
Student-centered, values teacher agency, and may or may not use technology | Data-driven, relies on digital twin technology, and enables data flows beyond the classroom, contributing to an audit culture |
Intimate knowledge of what the student is capable of and how much they can be challenged (Zones of Proximal Development) | Real-time data knowledge limits how and what students are challenged on |
Has existed for decades (e.g., enrichment for gifted students, clinics for struggling students) | Newer phenomenon with AI tutors that personalise learning through algorithmic adjustments |
Relies heavily on teacher-student relationships | Relies heavily on data-driven insights |
‘Foie Gras’ of education (carefully crafted, high quality) | ‘Fast Food’ of education ( Thornton et al. (2016)) (templated, mass-produced) |
A high-quality teacher can stretch and challenge students based on individual learning preferences | Digital technology has fixed, templated delivery (i.e., content is structured based on coding constraints) |
Face-to-face (including online tutoring) | Adaptable to Metaverse and AI-driven learning environments |
Dependent on teacher availability and curriculum delivery timelines | Allows students to ‘learn at their own pace,’ independent of teachers |
Becomes a privilege of the wealthy (one-on-one tutoring is costly) | Scalable and templatable for mass audiences |
Success is holistic and individualised | Success is measured by data and return on investment (ROI) |
The Case Against Personalised Learning
Given the transformative potential of personalised learning (be it human-led) or technology driven personalisation, why would anyone argue against either?
1. Cost and Accessibility
Personalised learning is expensive. As my own experience with my children, the cost of a Maths tutor for my 7 year old daughter is £65 per hour! An AI personalised tutor that keeps coming up on my Facebook feeds called Synthesis charges $300 per year for one child (I have 3 children). This will turn out to be quite an expensive tutor for one child, per subject, per year. However, it clearly is no where near as expensive as a human tutor.
As Pelletier (2024) argues, the drive towards AI-based personalisation is not about improving learning, but about expanding markets. Big Tech firms, through EdTech solutions, gain access to student data while embedding themselves into the education sector (Williamson 2022).
2. The Tension Between Individual vs. Mass Education
While personalised learning focuses on the individual, education systems operate at scale. The amount of time and preparation required for one-on-one learning makes it inefficient for large classrooms. In developing countries, personalised learning is virtually non-existent due to the financial and structural barriers. Countries like China and India often have 40-60 students in one class where personalised learning would be impossible for teachers. One could argue that some of the best and brightest minds in our world have come from these countries. Teachers must prepare bespoke materials, track individual progress, and offer tailored support—all of which are resource-intensive. In contrast, AI-driven personalisation offers pre-set templates, allowing for mass adaptation with minimal human oversight.
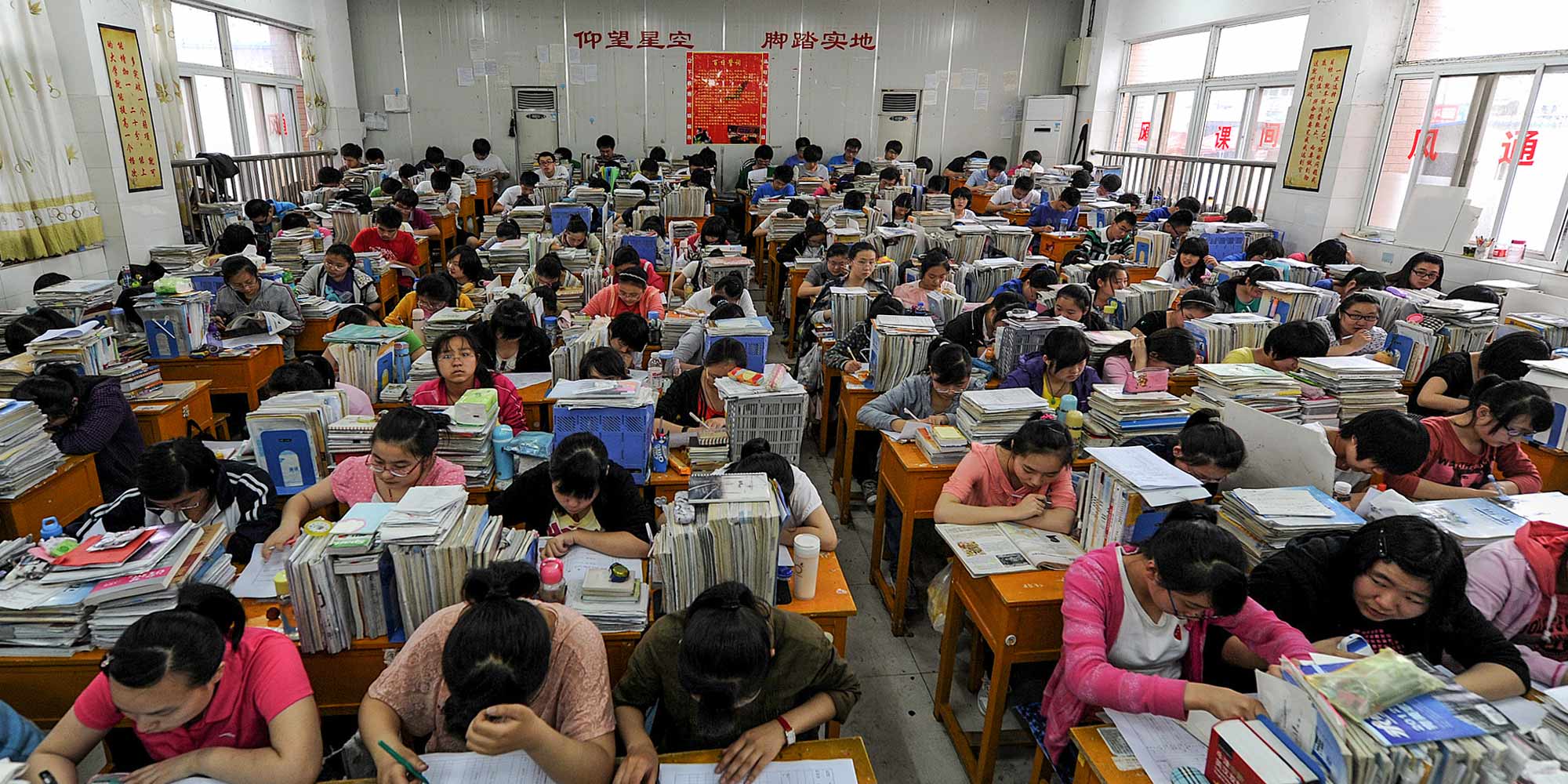
https://www.sixthtone.com/news/1000089
The problem is not just efficiency, but ideology. Education, as Biesta (2021) argues, serves three functions:
- Qualification (gaining knowledge and skills),
- Socialisation (becoming part of a community),
- Subjectification (developing as a unique individual).
Personalisation through AI is solid at qualification, but weak at socialisation and subjectification. If education is only about measurable outputs, what happens to the aspects of learning that can’t be quantified ?
3. The Charisma of Digital Education and its Divide
The introduction of digital twin technology and AI personalisation exacerbates inequalities and is misleading. Even identical twins aren’t identical, so how can we say that a human has a digital twin? No AI will ever replicate or even fathom the experience of the human student which must be taken into account when learning. Personalised learning systems claim to adapt to the individual and their needs, but if they work from incomplete data ie. the humaness of what has brought them to this point of learning on that day, they may be shaping and constraining the student rather than truly mirroring them. This can cause a series of errors:
- Bias & Misrepresentation: The digital twin only “knows” what it has been told—meaning biases in data and algorithmic decision-making can create a distorted view of a student.
Image: https://mind.help/
- Feedback Loops and Zones of Proximal Development : If a system predicts a student’s capabilities based on past data, does it actually limit their potential by reinforcing past weaknesses instead of allowing for transformation? Will it ever really push the student and who will decide what and how far that push will be? No one.
- Which is in control?: If the digital twin begins to shape educational experiences and becomes the judge of how far to challenge the student, who is really in control—the student or the system (it certainly won’t be the teacher)? And if students are shaped by algorithms, what happens to their agency in learning?
4. Never Letting Go
The Gradual Release of Responsibility model (Pearson & Gallagher, 1983) is built on the idea that learning progresses from teacher-led instruction to student independence. However, AI-based personalisation disrupts this progression because:
-
Perpetual Scaffolding – AI systems are designed to provide continuous, adaptive support, but they may never step back and allow the student to struggle, reflect, and internalise learning independently. Instead of fostering autonomy, AI risks creating long-term dependence.

Image: University of Florida
-
Overreliance on External Guidance – If an AI is constantly optimising the learning path and suggesting answers, students may not develop the critical thinking and problem-solving skills needed to navigate uncertainty and make independent decisions (see Bloom’s Taxonomy below).
-
Reduction of Metacognitive Growth – A key part of learning is developing self-regulation skills—knowing how to learn. AI-driven personalisation, by continuously guiding the learner, may deprive students of opportunities to set their own goals, monitor their understanding, and reflect on their learning process.
-
Risk of Passive Learning – Instead of actively constructing knowledge and making cognitive leaps, students may become passive recipients of AI-curated content, which limits their ability to engage in deep, self-directed learning.
Writing—in the classroom, in your journal, in a memo at work—is a way of bringing order to our thinking or of breaking apart that order as we challenge our ideas. If a machine is doing the writing, then we are not doing the thinking.
– Jane Rosenzweig, director of Harvard College’s Writing Center
The fundamental problem is that AI-based personalisation may never truly “let go”, contradicting the core principle of effective pedagogy: enabling students to think for themselves. If AI systems take over too much of the learning process, they risk producing students who are excellent at following recommendations but struggle to generate their own insights, think critically, or learn independently.
5. The Dehumanisation of Teaching & Learning
The increasing reliance on technology-led personalisation is already eroding teacher autonomy. Teachers are being evaluated based on data analytics, rather than holistic teaching skills. How a teacher makes a child feel, empowers them, and builds their confidence—none of this is measurable in data-driven models. In fact, a teacher has more insight into Maslow’s hierarchy of the student’s needs and how ‘prepared’ they are to learn that day. They will be automatically adjust for nuances of the student’s behaviour.
:max_bytes(150000):strip_icc():format(webp)/4136760-article-what-is-maslows-hierarchy-of-needs-5a97179aeb97de003668392e.png)
Maslow’s hierarchy of needs ‘A Theory of Human Motivation’ 1943
Pelletier (2024) warns that AI-driven education is a one-way discourse—students interact with algorithms, not human educators, who understands them as an individual. Teachers push back against AI tutors, but with little success, as schools adopt these tools for efficiency and marketing.
Big Tech’s role in education is ideological as much as technological. The argument that “AI will lighten teacher workloads” has existed for decades, yet evidence remains limited. Instead, AI circumvents teachers, rather than assisting them. The classroom becomes a site for data extraction, with teachers blamed when AI systems underperform.
Conclusion: The Future of Personalised Learning
The quandry of personalised learning is that while it is the ideal, it is not universally achievable. Human-led personalised learning is expensive, resource-intensive, and reserved for the privileged. AI-led personalisation, while scalable, does not and cannot replicate the relational depth of teacher-student interaction.
As Biesta (2021) reminds us, education is not just about efficiency—it is about interruption, suspension, and sustenance. Interruption occurs when students encounter new ideas that challenge them. Suspension allows for reflection and exploration beyond standardised outcomes. Sustenance refers to the relational support provided by educators, which AI cannot replace.
If personalised learning is to be truly transformative, we must:
- Ensure equitable access: Not just for the wealthy, but for all students.
- Balance AI integration with teacher agency: AI should support, not replace teachers.
- Reclaim education from Big Tech: Ensure student data remains protected and educational decisions are made by educators, not algorithms.
Without these safeguards, education risks becoming a privatised, automated system, where students are merely data points and teachers are reduced to facilitators of AI-driven learning.
____________________________________________________________________________________________________
References
Arantes, J. (2023) ‘Digital twins and the terminology of “personalization” or “personalized learning” in educational policy: A discussion paper’, Policy Futures in Education, 22(4), pp. 524-543. Available at: https://doi.org/10.1177/14782103231176357
https://assets.publishing.service.gov.uk/media/66bdc2de3effd5b79ba490fd/Special_educational_needs_and_disability_analysis_and_summary_of_data_sources_Aug24.pdf
Williamson, B., & Komljenovic, J. (2022). Investing in imagined digital futures: The techno-financial ‘futuring’ of edtech investors in higher education.Critical Studies in Education: https://doi.org/10.1080/17508487.2022.2081587.
Thornton LE, Lamb KE, Ball K (2016) Fast food restaurant locations according to socioeconomic disadvantage, urban–regional locality, and schools within Victoria, Australia. SSM – Population Health 2: 1–9. Crossref. PubMed.
https://citt.ufl.edu/resources/the-learning-process/designing-the-learning-experience/blooms-taxonomy/
Hello Nishel,
This is a wonderful artefact that highlights the critical debates for and against personalisation and personalised learning, while also linking us back to the purposes of education beyond efficiency, such broad purposes like interruption, suspension, and sustenance. With this multimodal blog you have clearly cited and utilised literature and infographics to advance creative critique and debate on why anyone would argue against personalised learning, despite its promised benefits of efficiency, adaptability, and customized learning experiences. Good use of the course reading resources and from your own searches to enrich the work.
I would add that, the conflation of these terms “personalisation and personalised learning” in educational policy and EdTech marketing discourse, provides data-driven, technology-led ‘personalization’ with an undue advantage over human-led ‘personalized learning. This is turn has had a significant impact on the role of the teacher in education.
I note these your constructive remarks that are positively anticipatory……as you indicate that,
“If personalised learning is to be truly transformative, we must:
Ensure equitable access: Not just for the wealthy, but for all students; Balance AI integration with teacher agency: AI should support, not replace teachers; Reclaim education from Big Tech: Ensure student data remains protected and educational decisions are made by educators, not algorithms;
Without these safeguards, education risks becoming a privatised, automated system, where students are merely data points and teachers are reduced to facilitators of AI-driven learning”.
And also following on from what we discussed in the tutorial, We need to develop consensus on what personalised learning is and could be. Is AI-led personalisation for enabling scaling? Or for personalising learning experiences as progressively organised in the curriculum frameworks? Or as earlier advanced by Dewey .. personalised education. What aspects of the teacher-led method/s we wish to build upon, how to mitigate the bias that is likely to arise from scaling or (dis)advantage, What frameworks and good practice guides are required to support this, and what data and educational control (if any) we are willing to cede to third-party interests. Does Ed-Tech personalised learning cater for the already intrinsically motivated learner?
With this blog, you are already moving to the next theme of reclaiming the future, and looking forward to the preferable future you will collaboratively envision and create.