Early Big Data and AI Algorithmic Bias
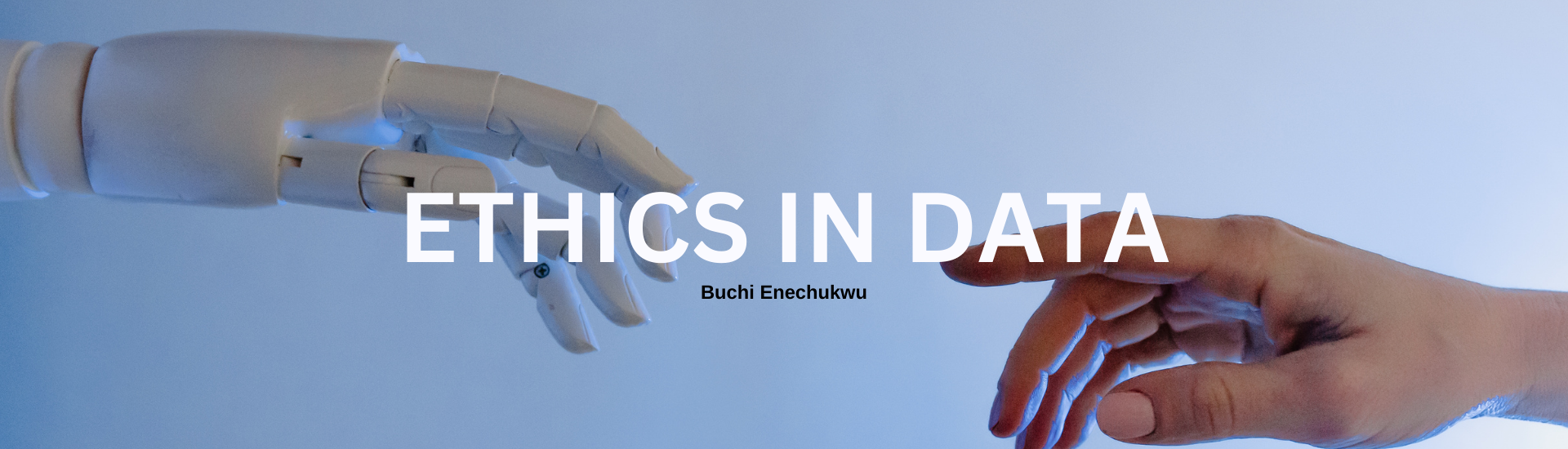
The course module, Ethical Data Futures, offered a fascinating exploration of the ethical complexities surrounding data handling. It exposed the pitfalls of data management, motivating a deeper understanding of this space. While reading the Excavating AI article, one of the essential readings, I caught a glimpse into the historical foundations of today’s AI. The authors’ emphasis on the diverse political perspectives present at the dawn of AI development resonated strongly through the analysis of Imagenet imagery as inherently political, which was particularly insightful. It went beyond the simple question of finite versus infinite data, revealing the biases embedded in mislabeled images, especially those concerning people.
While I paid attention to Professor Vallor’s analysis, it further illuminated this politicisation of data. Previously, I viewed data labeling as solely a descriptive process. However, her perspective challenged this assumption. She revealed that the very act of sourcing images and data from the early internet (2009) reflected the elitist demographics of major internet users at the time. Her point about the exclusion of those without internet access deeply resonated with me because I thought it effectively silenced a significant portion of the population. A particularly disturbing aspect, highlighted in both readings and discussions, was the inappropriate labeling of people in the images. Using labels like “slut” or “loser” is not only inaccurate but also sends a damaging message. It raises the question of how someone’s personality could possibly be discerned from a single image. These labeling practices speak volumes about the values and qualifications of those involved in services like Amazon Mechanical Turk and the foundation on which AI is built. The focus on speed and profit, with little regard for accuracy or sensitivity, paints a concerning picture.
While Professor Vallor opined that the labelers might be from low-income countries, I respectfully disagree. Accessibility limitations would have likely restricted participation in many such countries. For instance, with the use of Amazon Mechanical Turk, people in underdeveloped countries do not have access. Priority are likely been given to users in the US, reflecting the dominant demographic for labeling tasks. Furthermore, internet access in low-income countries during this era would have been limited, further restricting participation. Therefore, I argue that the labelers likely originated from a similar cultural background, potentially sharing similar biases prevalent in their communities. This lack of diversity further exacerbates the ethical issues.
The underrepresentation during AI development creates vulnerabilities for political manipulation and the misrepresentation of certain communities. This constitutes a significant ethical and political bias. To understand and counteract these biases, the course included further readings, group discussions, and a culminating case study reflection.
Reference
(Data)