Now, imagine that this tapestry, with its shimmering patterns and ugly knots, is being reflected through a machine-built mirror: a Large Language Model (the thing behind this AI Hub Bub).
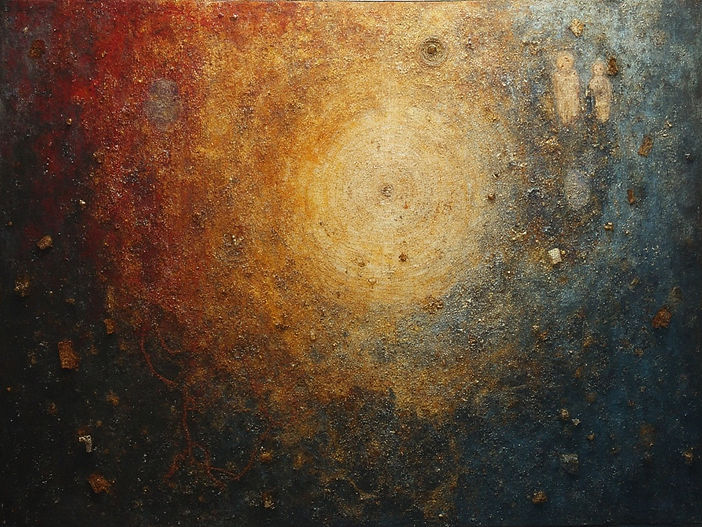
The image above is one version of that.
The art Generated from a model based on just that.
However, its more than just images and colors.
These models, trained on massive troves of text from the internet, ingest the moral fibers of countless authors, cultures, and time periods.
But do they present these moral strands faithfully, or do they distort them like a carnival mirror?
In many ways, LLMs serve as a powerful metaphor for the state of our own moral conversation. We live in a world rife with fragmented values.
Alasdair MacIntyre, the Scottish philosopher, observed that we struggle to find common ground on what constitutes “the good life” or a virtuous society.
Instead of a shared moral compass, we have multiple moral vocabularies all talking past one another. It’s as if we’re reading from different dictionaries, defining words like “justice” or “freedom” through our own cultural, political, or personal lenses.
The moral fragmentation MacIntyre described isn’t just an abstract problem—it’s etched into the everyday language we use, the political debates we witness, and the social media posts that flood our screens.
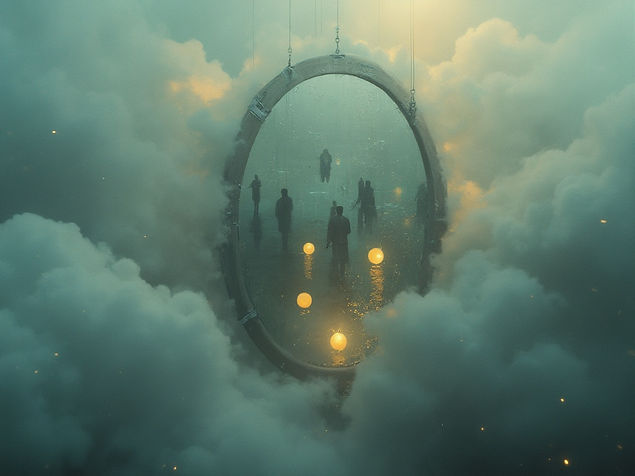
As LLMs learn from these texts, they inevitably pick up on this fragmentation.
They will “see” how certain words are used differently by different groups.
How “loyalty” or “fairness” can carry entirely distinct connotations in different cultural contexts.
One approach to grappling with these moral complexities is through what psychologists and social scientists call Moral Foundations Theory (MFT).
MFT suggests that our moral thinking can be understood in terms of a handful of core values or “foundations”: Care versus Harm, Fairness versus Cheating, Loyalty versus Betrayal, Authority versus Subversion, and Sanctity versus Degradation.
Even if we don’t all agree on how these should play out in practice, these categories help us describe and analyze moral disagreements.
So, what happens when these foundational values—these building blocks of moral thought—intersect with advanced language models?
On one hand, LLMs can reflect back to us the diverse moral landscapes they’ve absorbed. By analyzing their outputs, we can spot patterns: Do they tend to emphasize Authority more when discussing governance? Are they more likely to invoke Care or Harm when prompted about social policy?
In other words, the model’s “moral profile” might shine a light on the moral patterns hidden in the data it learned from.
However, simply counting words or tallying mentions of certain moral concepts doesn’t fully capture the complexity of how we think and talk about values.
This is where newer techniques come into play. One particularly promising approach is called Contextualized Construct Representation (CCR).

Without getting too lost in the technical weeds, CCR essentially lets us map psychological scales—like those from MFT or other ideological frameworks—onto modern language models.
Instead of just measuring how often a model uses certain moral words, CCR helps us interpret moral concepts in context, taking into account how a model “understands” a concept’s meaning across different sentences, themes, and nuances.
Why does this matter?
Well, language models might not have beliefs or intentions the way humans do, but their outputs can influence our perceptions. If we rely on them for summaries, analyses, or advice, their embedded moral tendencies can shape the kind of information and perspectives we encounter.
Consider a model that, due to its training data, subtly frames discussions of immigration policy around authority and loyalty rather than fairness or care. Over time, this could nudge the conversation, emphasizing certain moral values while downplaying others—effectively contributing to our real-world echo chambers.
Of course, LLMs don’t come with a pre-installed moral compass. Their creators can put guidelines in place—some might heavily moderate outputs to avoid hate speech or misinformation, others might err on the side of open-ended “neutrality.”
But each decision about what’s allowed and what’s restricted encodes a moral choice. Different companies adopt different standards, and the models they produce may reflect these moral ecosystems.
Much like the societies MacIntyre described, the AI landscape is not unified in moral direction. Instead, it’s a patchwork of moral assumptions and institutional choices.

By using methods like MFT-based analysis and CCR, researchers aim to break down the complexity of how LLMs handle moral language.
Rather than seeing AI as a monolithic black box, we can start to map its moral terrain.
We can say:
“In this domain, the model’s language skews toward emphasizing fairness. In that domain, it leans more heavily into authority-based reasoning.”
As we refine these techniques, we gain a clearer picture of how moral ideas are distributed across the model’s outputs.
This isn’t just an academic exercise. Understanding the moral profiles of LLMs can help developers build more transparent and accountable systems.
Policymakers might use these insights to guide regulations that prevent AI from systematically marginalizing certain viewpoints. Ethicists and social scientists can engage more productively with the models, knowing where to look for potential biases or moral blind spots.
And everyday users, though they might not dig into the technical details, benefit from increased awareness about the forces shaping the information they receive.
We’re still in the early days of translating philosophical insight, psychological theory, and computational sophistication into practical tools for understanding GenAI’s moral dimension.
But the direction is clear. The more we learn about how moral concepts are embedded in these language models, the better equipped we are to ensure that AI doesn’t just mirror our existing moral chaos, but perhaps helps us see it more clearly—and maybe even inspire us to strive for greater moral coherence.
In other words, by studying how machines “understand” morality, we’re holding up that mirror and scrutinizing the tapestry it reflects.
What we find there might not always be comforting—it may highlight the divisions and disagreements we’d rather ignore.
But shining a light on these fractures is the first step toward grappling with them.
In this sense, LLMs offer not only a challenge but an opportunity: they remind us that our moral world is complicated, often contradictory, and in desperate need of careful, critical reflection.
