Interdisciplinary Data Science for Social Good
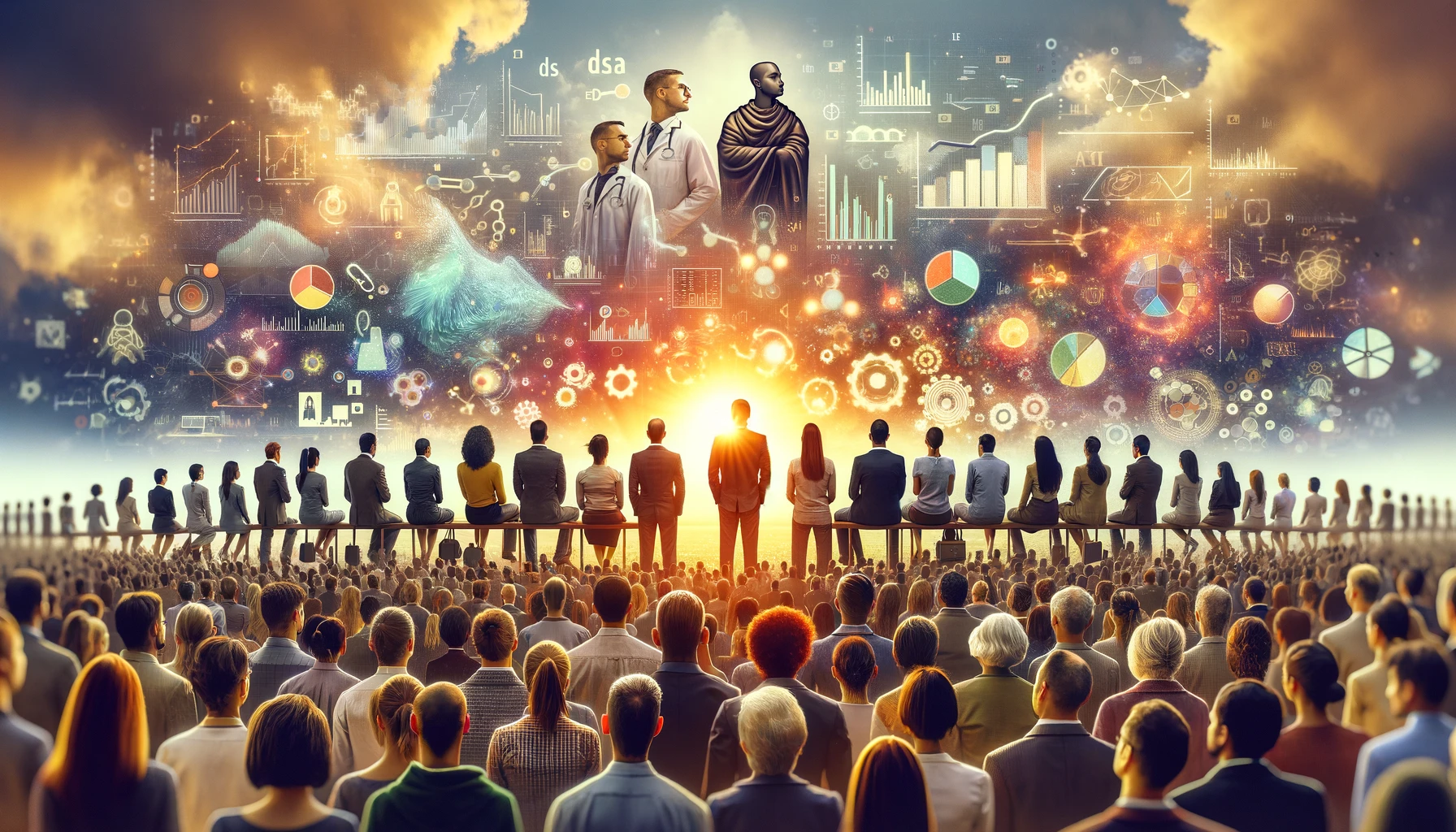
Introduction
In an increasingly complex world, where social issues are interwoven with global narratives and diverse perspectives, interdisciplinary approaches have become crucial in understanding and addressing these challenges. Interdisciplinary studies, a fusion of various fields like data science, history, literature, and more, provide a rich tapestry of insights and methodologies to tackle societal issues. Through my journey in interdisciplinary studies and data science for society courses, my perspective has broadened significantly, allowing a more holistic view of how different disciplines intersect and contribute to understanding complex social dynamics.
Data science, in particular, plays a pivotal role in this interdisciplinary blend. It offers robust tools for analyzing and interpreting large datasets, identifying patterns and trends that might otherwise remain hidden. In the context of social justice, data science becomes a powerful ally. It helps in uncovering systemic inequalities, monitoring social changes, and evaluating the impact of policies aimed at addressing social injustices. By combining data science with insights from history, literature, and other fields, we can gain a more comprehensive understanding of the root causes and potential solutions to social injustices.
In this blog post, we will explore various interdisciplinary methods that combine data science with other disciplines to address and understand social justice issues. We will delve into case studies, including my work in using data science to facilitate civic action, to illustrate the potent combination of interdisciplinary approaches in creating meaningful change. The synergy of these diverse fields not only enriches our understanding but also empowers us to devise more effective strategies in the pursuit of a more equitable society.
The Need for Interdisciplinary Approaches
Social justice issues are inherently complex, often deeply rooted in historical, cultural, and socio-economic contexts that cannot be fully understood through a single-disciplinary lens. This complexity demands an interdisciplinary approach. Traditional single-discipline strategies, while effective in their own realms, may fall short when dealing with the multifaceted nature of social injustice. They can miss crucial nuances and connections that only emerge when multiple perspectives are combined.
In my experience, particularly through group projects in my interdisciplinary studies and data science for society courses, the value of diverse academic backgrounds becomes abundantly clear. Teams composed of students from various disciplines, including the humanities, social sciences, and technical fields, bring together a rich variety of perspectives. This diversity in thought and expertise not only enriches the discussion but also leads to more innovative and comprehensive solutions. For instance, a project may start with a data-driven analysis, but without the contextual understanding provided by history or the critical perspectives offered by literature and social sciences, the data alone might lead to misinterpretations or oversimplified conclusions.
The integration of data science with other fields like history, literature, and social sciences offers a powerful toolkit for understanding and addressing social injustices. Data science, with its capabilities in handling large datasets and uncovering patterns, offers a factual basis for understanding social phenomena. However, it is the historical context that provides the narrative behind these data, explaining why certain patterns exist. Literature, on the other hand, adds a humanistic touch, bringing in the human experiences and stories behind the numbers. Social sciences contribute by offering theories and frameworks to understand societal behaviors and institutions.
This interdisciplinary approach does not dilute the importance of each individual discipline. Instead, it enhances their strengths by filling in gaps that a single discipline might not address on its own. By acknowledging the complexity of social justice issues and leveraging the combined strengths of diverse fields, we can gain a more holistic and effective understanding, leading to more sustainable and impactful solutions.
Data Science in Social Justice: Theoretical Framework
The application of data science in social justice is grounded in a theoretical framework that intertwines the analytical power of data with the human-centric approach of social sciences. This framework leverages the strengths of data science — such as big data analytics, machine learning, and pattern recognition — to identify and analyze trends in social issues, while ensuring that the interpretations remain sensitive to the socio-cultural context.
In “Sustainable Knowledge: A Theory of Interdisciplinarity,” the concept of interdisciplinarity is explored as a means to bridge the gap between empirical data and the complex realities of human society. This approach is crucial in social justice work, as it allows for a more nuanced understanding of issues that are not readily apparent through quantitative data alone. By integrating insights from various disciplines, data science in social justice can avoid the pitfalls of reductionism and oversimplification.
“Inventive Methods: The Happening of the Social” further expands on this by highlighting the need for inventive, non-traditional methods in understanding social phenomena. Data science, when combined with creative methodologies from other disciplines, can uncover hidden narratives and give voice to marginalized communities often left out of mainstream data analysis. This aligns with the core goals of social justice, which seek to elevate the experiences and challenges of underrepresented groups.
“Introduction to Interdisciplinary Studies” emphasizes the importance of collaboration across disciplines to address complex societal issues. In the context of social justice, this means incorporating the ethical, cultural, and historical perspectives alongside data-driven insights. Data science provides the tools to quantify and analyze social inequalities, but it is the interdisciplinary framework that contextualizes these findings within the broader spectrum of human experience.
Ultimately, the theoretical framework for using data science in social justice is one that advocates for a balanced approach. It recognizes the power of data in uncovering trends and patterns in social issues, but also acknowledges the necessity of incorporating diverse perspectives to fully understand and address these issues. This holistic approach ensures that data science serves as a tool for empowerment and change, rather than merely a technical exercise.
Public Good Software (PGS)
I previously worked for Public Good Software (PGS) which serves as an exemplary case study for the intersection of data science and social justice. PGS has developed a technology platform that uses data science to match news content with relevant social causes. This innovative approach not only highlights the potential of data science in addressing social issues but also underscores the importance of an interdisciplinary approach in technology development.
At the core of PGS’s technology is the use of text-matching, natural language processing, and machine learning to efficiently pair news articles with relevant social causes. This system addresses the challenge of manual content analysis, which is slow, inconsistent, and labor-intensive. By automating the process, PGS effectively connects readers, publishers, NGOs, and brands with social causes, encouraging actions like fundraising, advocacy, and education.
PGS’s journey began with a simple ‘Take Action Button’ on news sites, which evolved into a more sophisticated system integrating a custom taxonomy of 27 social causes. This evolution was marked by a transition from manual processes to automated, machine learning-based solutions. The PGS team developed a taxonomy inspired by the UN Sustainable Development Goals, refined to better match news stories with social causes.
PGS’s platform has had a significant impact on facilitating civic action and engagement. By making news content actionable, PGS provides insights into consumer interests in social causes, helping policymakers, brands, and NGOs align their efforts with public concerns. This actionable approach to news content has empowered readers to participate more actively in social causes, bridging the gap between awareness and action.
Despite its successes, PGS faced challenges such as matching accuracy and scalability. The initial machine learning classifier, based on the Naive Bayes algorithm, had limitations in accuracy and categorization. As the platform scaled, balancing the accuracy of matches with the growing volume of content and diversity of social causes presented further challenges. The team worked on refining their classification models and improving their taxonomy to better align with real-world social causes. Quality assurance processes were implemented to ensure the relevancy and accuracy of matches, illustrating the ongoing need for human oversight in automated systems.
The Public Good Software (PGS) model showcases a pioneering approach in applying data science to social justice, offering valuable insights for broader applications. This model’s success in aligning news content with relevant social causes through advanced data processing techniques can be transformative in various fields beyond its current scope.
In policy-making, the PGS model’s ability to analyze and synthesize large datasets can inform more nuanced and effective policies. By identifying and understanding complex patterns within social issues, policymakers can develop targeted strategies that address the root causes of societal challenges, rather than just the symptoms. This data-driven approach ensures that policies are grounded in reality, making them more likely to succeed in creating positive change.
For non-governmental organizations (NGOs), the PGS approach can revolutionize how they address social issues. By leveraging data science, NGOs can gain deeper insights into the needs and challenges of the communities they serve. This enables them to allocate resources more efficiently, design more impactful programs, and measure the effectiveness of their initiatives in real-time. The ability to rapidly adapt to changing circumstances based on data-driven feedback is crucial for NGOs operating in dynamic social environments.
Lastly, in raising social awareness, the PGS model demonstrates the power of technology in engaging the public. By making social issues more relatable and actionable through data visualization and storytelling, this approach can amplify the voices of marginalized communities and foster a more informed and empathetic public discourse. It encourages active participation and advocacy, bridging the gap between awareness and action.
Conclusion
The intersection of interdisciplinary studies and data science plays a crucial role in understanding and addressing complex social issues. By combining insights from diverse fields like history, literature, and social sciences with the analytical power of data science, we can gain a more comprehensive understanding of social dynamics and develop effective strategies to address social injustices.
Throughout this post, we have explored various interdisciplinary methods and case studies, highlighting the power of interdisciplinary approaches in creating meaningful change. The use of data science in Public Good Software (PGS) exemplifies how interdisciplinary collaboration can lead to innovative solutions in the pursuit of social justice. The theoretical framework for using data science in social justice emphasizes the importance of balancing data analysis with diverse perspectives and contextual understanding.
As we continue to leverage data science and interdisciplinary approaches in addressing social challenges, it is crucial to maintain an ethical and holistic perspective. By considering the cultural, historical, and social contexts within which data operates, we can ensure that our efforts towards social justice are sustainable and inclusive.
Interdisciplinary data science offers a transformative pathway towards a more equitable society. By embracing diverse academic backgrounds, incorporating contextual understanding, and using data-driven insights responsibly, we can empower marginalized communities, raise social awareness, and advocate for positive change. Let us continue to harness the power of interdisciplinary collaboration and data science to create a more just and equitable world.
References
Robert Frodeman (2013) Sustainable knowledge a theory of interdisciplinarity. Basingstoke, Palgrave Macmillan.
Celia Lury & Nina Wakeford (Eds.) (2012) Inventive Methods: The Happening of the Social. London, Routledge. doi:10.4324/9780203854921.
Kingery, E., Mccallum, Q. and Brown, S. (2019). Using Data Science to Facilitate Civic Action. Bloomberg Data for Good Exchange.
Allen F. Repko, R.S. & Michelle Phillips Buchberger (2020) Introduction to interdisciplinary studies. Third edition. Los Angeles, SAGE.