- Smallville Revisited: How the 25-Agent Experiment Was Trained
Back in 2023, Joon Sung Park and colleagues unleashed 25 generative agents into a pixel town [1] that looked suspiciously like The Sims.
Training recipe: Each agent started with a single-sentence biography (“Evelyn, the friendly barista who loves art”) and the weights of a large-language model (LLM). The researchers did not pre-script behaviour. Instead, they wired the LLM to a cognitive loop—perception → memory → retrieval → reflection → planning → action. As the agents sensed events (e.g., seeing a neighbour), they stored that observation as short-term memory, later retrieving, summarising, and planning the next move. A few dozen such cycles per in-game day were enough for one agent to propose a Valentine’s Day party; the other 24 autonomously spread the news, formed dates, baked cupcakes, and showed up on time.
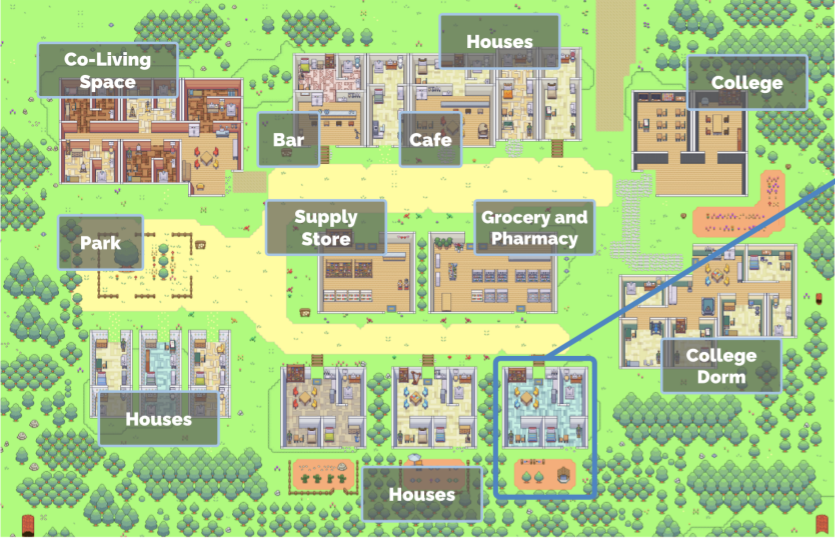
- Bigville: Scaling Up to 1,052 AI Doppelgängers
If Smallville was a sitcom cast, Stanford’s follow-up—“Generative Agent Simulations of 1,000 People”—is a demographic thriller.
How they trained it? The team first recruited 1,052 U.S. adults. Each participant completed a two-hour, LLM-mediated interview, producing roughly 6,500 words of life stories, beliefs, preferences, and experiences. Four specialised “virtual PhDs” (a psychologist, economist, political scientist, and demographer) then summarised each transcript into bullet-point reflections so the agent could reason beyond raw dialogue. Finally, those memories were piped into the same cognitive loop used in Smallville, yielding 1,052 unique agents [2].
Does it work?
To find out whether the AI “twins” really behaved like their flesh-and-blood counterparts, the researchers ran both groups through the same social-science obstacle course. First came the General Social Survey (GSS), a gold-standard questionnaire covering everything from civic values to favourite TV genres; the agents reproduced roughly 85 percent of their owners’ answers, almost as close as the humans matched themselves two weeks later. Next was the Big-Five personality inventory, where scores for openness, conscientiousness, extraversion, agreeableness, and neuroticism lined up almost one-for-one with the originals. Finally, in the Dictator Games—behavioural-economics mini-games that probe fairness and generosity—the AI players offered and accepted splits that differed from their humans by only a few cents on average. In short, the digital doppelgängers didn’t just talk like their humans; they shared their survey opinions, personality fingerprints, and moral instincts as well [2].
Why add 1,027 more agents? With a thousand-plus personalities, researchers can now prototype policy changes, marketing campaigns, or public-health messages across a population-scale sandbox before risking real-world.
- Why This Leap Matters
Early agent-based models (ABMs) showed that simple rule-following agents could recreate traffic jams, housing segregation, or stock-market bubbles [3]. By inserting LLM-powered memories onto those frameworks, generative agents now capture individual nuance—religious background, music taste, political leanings—at scale. That blend opens doors for:
- Evidence-driven policy rehearsal. Governments can test tax reforms inside Bigville first, spotting unrest long before protest banners hit Main Street.
- Organisational A/B tests. A business could test a four-day workweek on its virtual employees first, then instantly see whether people would quit less, feel happier, and get more work done.
- Interdisciplinary research. Economists, psychologists, and urban planners share one giant sandbox, accelerating cross-field hypotheses that were once impossible to coordinate [4].
- Ethical Seatbelts Required
Think of each AI twin as a super-bright mirror: it shows us a remarkably realistic reflection, but stare too long and you might get burned.
- Privacy first. Those two-hour interviews read like personal diaries—childhood stories, political views, heartbreaks. All that text must live in a locked digital vault with strict access logs, encryption, and clear rules about who can use it and why.
- Mind the bias. If most volunteers happen to be tech-savvy city folks, the agents will inherit that worldview and overlook rural or blue-collar perspectives. Researchers have to keep their sample diverse and run regular bias audits to catch blind spots early.
- Don’t give them a “soul.” The agents can sound weirdly human, but they’re still statistical models, not sentient beings. Policymakers and product designers must remember they’re talking to code, not a conscious citizen.
- Be transparent. Publish the data-handling practices, explain the model’s limits, and add big, friendly disclaimers when results feed into public decisions.
In short, lock up the personal data, double-check for hidden bias, and always label these twins for what they are—clever simulations, not living substitutes.
- Conclusion
In barely a year, generative agents jumped from a 25-person rom-com to a 1,052-person behavioural census. Smallville proved agents can plan parties; Bigville shows they can also answer surveys, negotiate ultimatum games, and debate politics with statistically believable accuracy. The next time someone proposes “testing it live,” you can reply, “Great—let’s spin up a thousand AI doppelgängers first.” Your future self (and perhaps your toaster) may thank you.
References
- Park, J. S., O’Brien, J. C., Cai, C. J., Morris, M. R., Liang, P., & Bernstein, M. S. (2023). Generative Agents: Interactive Simulacra of Human Behavior. UIST ’23 Proceedings.
- Park, J. S., Zou, C. Q., Shaw, A., Hill, B. M., Cai, C., Morris, M. R., Willer, R., Liang, P., & Bernstein, M. S. (2024). Generative Agent Simulations of 1,000 People. arXiv:2411.10109.
- Epstein, J. M., & Axtell, R. L. (1996). Growing Artificial Societies: Social Science from the Bottom Up. Brookings/MIT Press.
- Wooldridge, M. (2009). An Introduction to MultiAgent Systems (2nd ed.). Wiley.
https://hai.stanford.edu/news/computational-agents-exhibit-believable-humanlike-behavior
https://hai.stanford.edu/news/ai-agents-simulate-1052-individuals-personalities-with-impressive-accuracy